728x90
상황별 그래프 종류
- 비교 분석 : 막대차트, 라인차트 등
- 구성 분석 : 파이차트, 히트맵 등
- 관계 분석 : 스케터 차트 등
- 분포 분석 : 히스토그램, 박스플롯, 바이올린 플랏 등
데이터의 종류
- 연속형데이터 : 숫자, ex) 키, 몸무게, 판매량, 매출 ..
- 범주형데이터 : 범주 ex) 형액형, MBTI, 성별, 사는지역 …
- A사가 판매하는 10개의 제품의 월별 판매량 비교
- 비교 분석
- 막대(x: 범주형(월, 제품 명), y : 연속형(판매량)
- 각 항목별 판매량이 한눈에 확인할 수 있다.
- 높이 차이를 직관적으로 파악할 수 있다.
- 라인(x: 범주형(월), y : 연속형(제품에 따른 판매량)
- 월별 판매량 변화를 보기 쉬움
- 시간에따라 보기에 적합
- (기간이 길수록 라인이 괜찮을 것 같다.)
- 막대(x: 범주형(월, 제품 명), y : 연속형(판매량)
- 비교 분석
- 인구 밀도와 평균 수명 분석
- 관계 분석
- 스케터(x : 연속형, y : 연속형)
- 관계 분석
- 학생들의 시험 점수 분석
- 분포분석
- 히스토그램(X or y : 연속형(학생 시험점수))
- 바이올린(X or y : 연속형(학생 시험점수))
- 분포분석
- 대륙(아시아, 유럽,…)의 연간 GDP 분석
- 관계 분석 (X: 연도, Y : GDP, 색상 : 대륙, 원크기 : GDP)
- 스케터 : 각 대륙별로 나라를 x축에 넣고 다른 색으로 표시해주어 분포를 알 수 있게 해줌
- 비교 분석
- 막대(비교)
- 대륙별이어서 막대로 해도 한눈에 보기 쉬울거같아서했습니다
- 대륙으로 카테고리 나누고 그 사이에서 높은 순서대로 막대그래프를 하면 비교분석하기 편할 것 같아서요
- 라인(추세)
- 대륙별 GDP 추세 변화를 파악하려는 의도
- 막대(비교)
- 구성 분석
- 히트맵(비교)
- 나라가 많으니까 높은 GDP는 찐한 색, 낮은 GDP는 연한 색으로 표시할 수 있으면 보기 편할 것 같아서 히트맵
- 파이차트(몇개년 안될 때)
- 히트맵(비교)
- 지도 : 지도위에 히트맵으로 표현하면 직관적으로 데이터를 파악할 수 있을거 같습니다.
- 관계 분석 (X: 연도, Y : GDP, 색상 : 대륙, 원크기 : GDP)
Colab vscode 한글 환경설정
In [ ]:
# # colab
# # 런타임 다시시작을 해야 한글폰트가 적용 됩니다.
# !sudo apt-get install -y fonts-nanum
# !sudo fc-cache -fv
# !rm ~/.cache/matplotlib -rf
In [ ]:
# vscdoe
#패키지를 불러옵니다.
import matplotlib as mpl
import platform
#주피터 노트북내에 그림을 표시 합니다.
%matplotlib inline
my_platform = platform.system()
# 폰트 설정
if my_platform == 'Linux':
mpl.rc('font', family='NanumBarunGothic')
elif my_platform == 'Windows':
mpl.rc('font', family='Malgun Gothic')
elif my_platform == 'Darwin':
mpl.rc('font', family='AppleGothic')
#그래프의 폰트를 선명하게 출력합니다.
from IPython.display import set_matplotlib_formats
set_matplotlib_formats('retina')
# 그래프 음수값 깨짐 방지
mpl.rc('axes', unicode_minus=False)
/var/folders/lq/nqfbjmpd3bl434m7j6ktxqn80000gn/T/ipykernel_55308/2568410305.py:20: DeprecationWarning:
`set_matplotlib_formats` is deprecated since IPython 7.23, directly use `matplotlib_inline.backend_inline.set_matplotlib_formats()`
데이터시각화(범주vs연속/matplotlib)
matplotlib
비교 분석
막대그래프
- X : 범주형 데이터
- Y : 연속형 데이터
In [ ]:
import matplotlib.pyplot as plt
fig, ax = plt.subplots()
fruits = ['apple', 'blueberry', 'cherry', 'orange']
counts = [40, 100, 30, 55]
bar_labels = ['red', 'blue', '_red', 'orange'] # _red : 이미 존재하는 red에 포함
bar_colors = ['tab:red', 'tab:blue', 'tab:red', 'tab:orange']
ax.bar(fruits, counts, label=bar_labels, color=bar_colors)
ax.set_ylabel('fruit supply')
ax.set_title('Fruit supply by kind and color')
ax.legend(title='Fruit color')
plt.show()
라인차트
- X : 연속형(시계열)
- Y : 연속형
In [ ]:
import matplotlib.pyplot as plt
import numpy as np
# Data for plotting
t = np.arange(0.0, 2.0, 0.01)
s = 1 + np.sin(2 * np.pi * t)
fig, ax = plt.subplots()
ax.plot(t, s)
ax.set(xlabel='time (s)', ylabel='voltage (mV)',
title='About as simple as it gets, folks')
plt.show()
구성분석
파이차트
X : 연속형
In [ ]:
import matplotlib.pyplot as plt
labels = ['Frogs', 'Hogs', 'Dogs', 'Logs']
sizes = [15, 30, 45, 10]
fig, ax = plt.subplots()
ax.pie(sizes, labels=labels)
Out[ ]:
([<matplotlib.patches.Wedge at 0x1427836a0>,
<matplotlib.patches.Wedge at 0x14288a7c0>,
<matplotlib.patches.Wedge at 0x142783cd0>,
<matplotlib.patches.Wedge at 0x142792190>],
[Text(0.9801071672559598, 0.4993895680663527, 'Frogs'),
Text(-0.33991877217145816, 1.046162142464278, 'Hogs'),
Text(-0.49938947630209474, -0.9801072140121813, 'Dogs'),
Text(1.0461621822461364, -0.3399186497354948, 'Logs')])
히트맵
- X : 범주형
- Y : 범주형
- value : 연속형
In [ ]:
import numpy as np
import matplotlib
import matplotlib as mpl
import matplotlib.pyplot as plt
vegetables = ["cucumber", "tomato", "lettuce", "asparagus",
"potato", "wheat", "barley"]
farmers = ["Farmer Joe", "Upland Bros.", "Smith Gardening",
"Agrifun", "Organiculture", "BioGoods Ltd.", "Cornylee Corp."]
harvest = np.array([[0.8, 2.4, 2.5, 3.9, 0.0, 4.0, 0.0],
[2.4, 0.0, 4.0, 1.0, 2.7, 0.0, 0.0],
[1.1, 2.4, 0.8, 4.3, 1.9, 4.4, 0.0],
[0.6, 0.0, 0.3, 0.0, 3.1, 0.0, 0.0],
[0.7, 1.7, 0.6, 2.6, 2.2, 6.2, 0.0],
[1.3, 1.2, 0.0, 0.0, 0.0, 3.2, 5.1],
[0.1, 2.0, 0.0, 1.4, 0.0, 1.9, 6.3]])
fig, ax = plt.subplots()
im = ax.imshow(harvest)
# Show all ticks and label them with the respective list entries
ax.set_xticks(np.arange(len(farmers)), labels=farmers)
ax.set_yticks(np.arange(len(vegetables)), labels=vegetables)
# Rotate the tick labels and set their alignment.
plt.setp(ax.get_xticklabels(), rotation=45, ha="right",
rotation_mode="anchor")
# Loop over data dimensions and create text annotations.
for i in range(len(vegetables)):
for j in range(len(farmers)):
text = ax.text(j, i, harvest[i, j],
ha="center", va="center", color="w")
ax.set_title("Harvest of local farmers (in tons/year)")
fig.tight_layout() # 재정렬
plt.show()
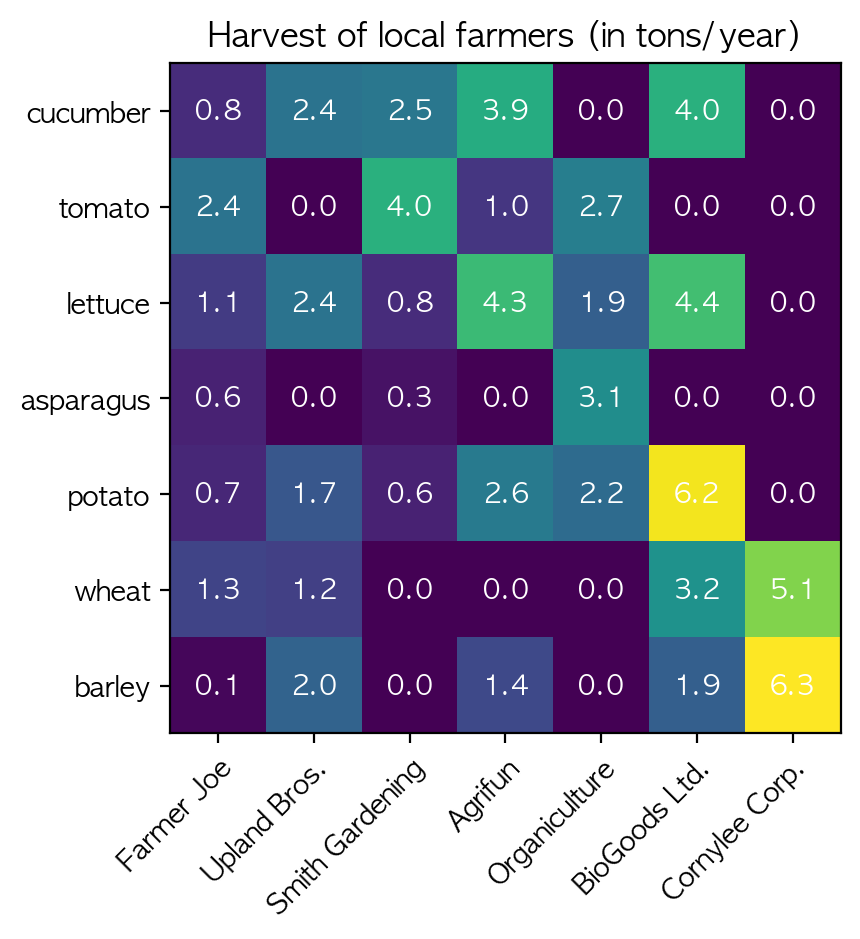
관계분석
스케터 차트
- X : 연속형
- Y : 연속형
- 원사이즈 : 연속형
In [ ]:
import numpy as np
import matplotlib.pyplot as plt
N = 50
x = np.random.rand(N) # 랜덤한 값 50개
y = np.random.rand(N) # 랜덤한 값 50개
colors = np.random.rand(N) # 랜덤한 값 50개
area = (30 * np.random.rand(N))**2 # 0 to 15 point radii
plt.scatter(x, y, s=area, c=colors, alpha=0.5) # s : 원 사이즈 , c : 색상, alpha : 투명도
plt.show()
분포 분석
히스토그램
- X, Y : 연속형
In [ ]:
import matplotlib.pyplot as plt
import numpy as np
from matplotlib import colors
from matplotlib.ticker import PercentFormatter
# Create a random number generator with a fixed seed for reproducibility
rng = np.random.default_rng(19680801)
N_points = 100000
n_bins = 20
# Generate two normal distributions
dist1 = rng.standard_normal(N_points) # 정규분포데이터 생성
fig, axs = plt.subplots()
# We can set the number of bins with the *bins* keyword argument.
axs.hist(dist1, bins=n_bins)
Out[ ]:
(array([3.0000e+00, 5.0000e+00, 5.9000e+01, 2.3900e+02, 8.3000e+02,
2.3340e+03, 5.2150e+03, 9.5590e+03, 1.4319e+04, 1.7675e+04,
1.7463e+04, 1.4353e+04, 9.5070e+03, 5.0910e+03, 2.1790e+03,
8.7000e+02, 2.3200e+02, 5.7000e+01, 8.0000e+00, 2.0000e+00]),
array([-4.54540983, -4.09007228, -3.63473474, -3.17939719, -2.72405964,
-2.2687221 , -1.81338455, -1.358047 , -0.90270946, -0.44737191,
0.00796564, 0.46330318, 0.91864073, 1.37397828, 1.82931582,
2.28465337, 2.73999092, 3.19532846, 3.65066601, 4.10600355,
4.5613411 ]),
<BarContainer object of 20 artists>)
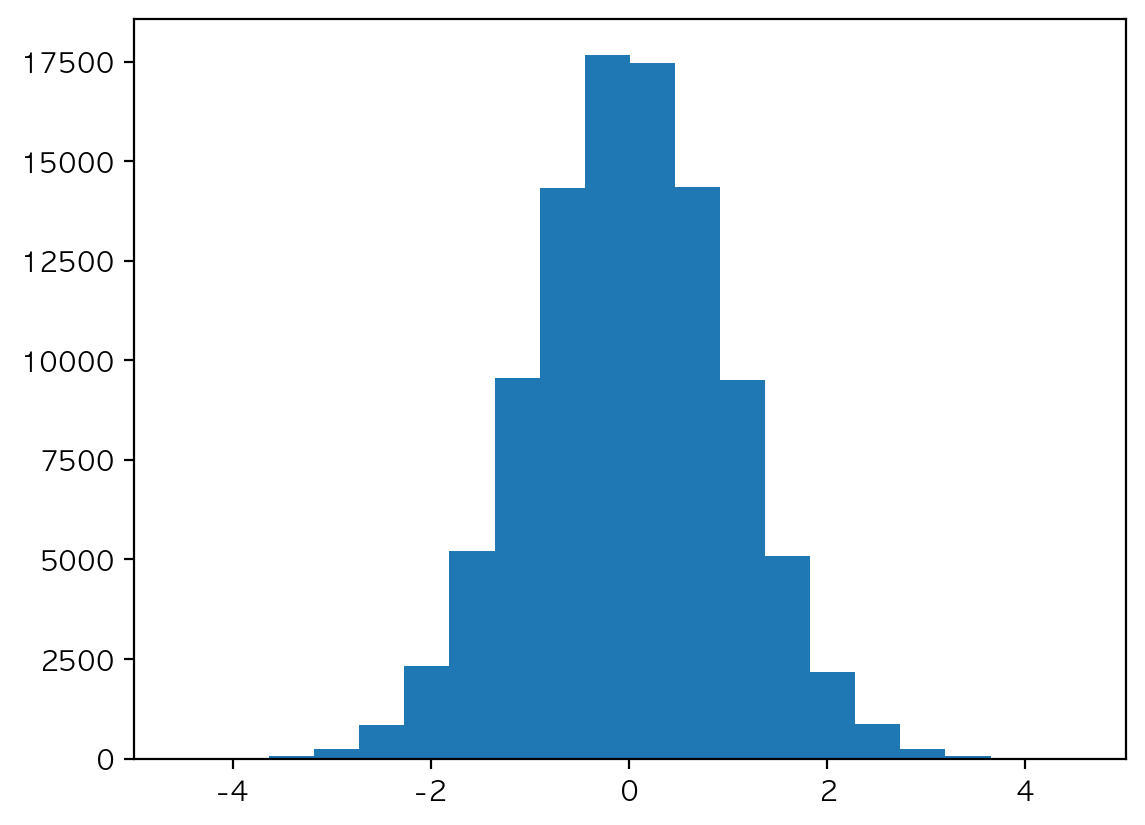
박스플롯, 바이올린 플롯
- X,Y : 연속형
In [ ]:
import matplotlib.pyplot as plt
import numpy as np
fig, axs = plt.subplots(nrows=1, ncols=2, figsize=(9, 4))
# 100개의 배열을 가지는 데이터 그룹 4개 생성
all_data = [np.random.normal(0, std, 100) for std in range(6, 10)]
# plot violin plot
axs[0].violinplot(all_data,
showmeans=False,
showmedians=True)
axs[0].set_title('Violin plot')
# plot box plot
axs[1].boxplot(all_data)
axs[1].set_title('Box plot')
plt.show()
matplotlib vs seaborn vs plotly
matplotlib
- 가장 먼저 생긴 시각화 라이브러리
- seaborn도 matplotlib 기반으로 만들어짐
- 그래프에 X,Y에 리스트(배열)로 넣어줘야 한다.
In [ ]:
import matplotlib.pyplot as plt
fig, ax = plt.subplots()
fruits = ['apple', 'blueberry', 'cherry', 'orange']
counts = [40, 100, 30, 55]
bar_labels = ['red', 'blue', '_red', 'orange'] # _red : 이미 존재하는 red에 포함
bar_colors = ['tab:red', 'tab:blue', 'tab:red', 'tab:orange']
ax.bar(fruits, counts, label=bar_labels, color=bar_colors)
ax.set_ylabel('fruit supply')
ax.set_title('Fruit supply by kind and color')
ax.legend(title='Fruit color')
plt.show()
seaborn
- matplotlib 기반으로 만들어졌다.
- pandas에서 활용하는 데이터프레임 데이터타입의 데이터를 시각화하는데 유리
In [ ]:
#!pip install seaborn
import seaborn as sns
df = sns.load_dataset("penguins")
df.head()
Out[ ]:
speciesislandbill_length_mmbill_depth_mmflipper_length_mmbody_mass_gsex01234
Adelie | Torgersen | 39.1 | 18.7 | 181.0 | 3750.0 | Male |
Adelie | Torgersen | 39.5 | 17.4 | 186.0 | 3800.0 | Female |
Adelie | Torgersen | 40.3 | 18.0 | 195.0 | 3250.0 | Female |
Adelie | Torgersen | NaN | NaN | NaN | NaN | NaN |
Adelie | Torgersen | 36.7 | 19.3 | 193.0 | 3450.0 | Female |
In [ ]:
g1 = df[['island', 'body_mass_g']].groupby('island').mean()
sns.barplot(data=g1, x=g1.index, y="body_mass_g")
Out[ ]:
<Axes: xlabel='island', ylabel='body_mass_g'>
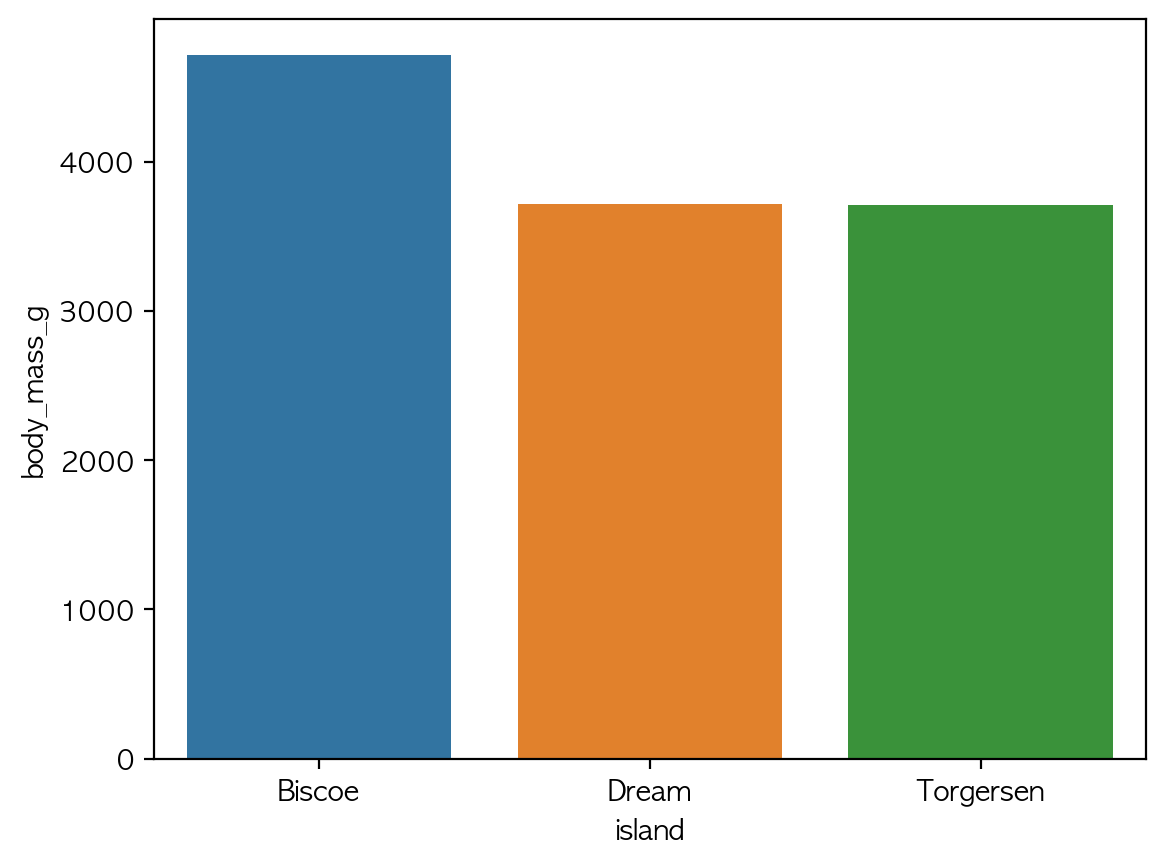
In [ ]:
sns.barplot(data=df, x="island", y="body_mass_g")
Out[ ]:
<Axes: xlabel='island', ylabel='body_mass_g'>
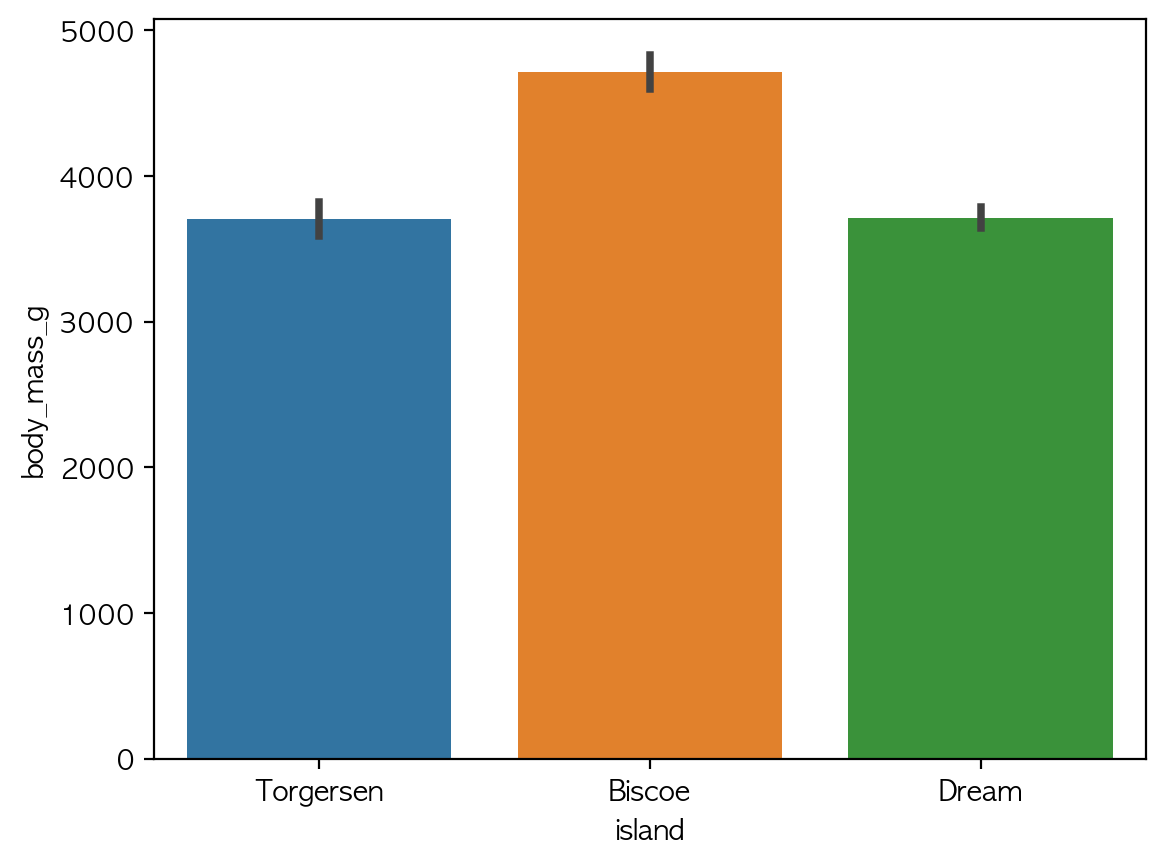
In [ ]:
sns.barplot(data=df, x="island", y="body_mass_g", hue="sex")
Out[ ]:
<Axes: xlabel='island', ylabel='body_mass_g'>
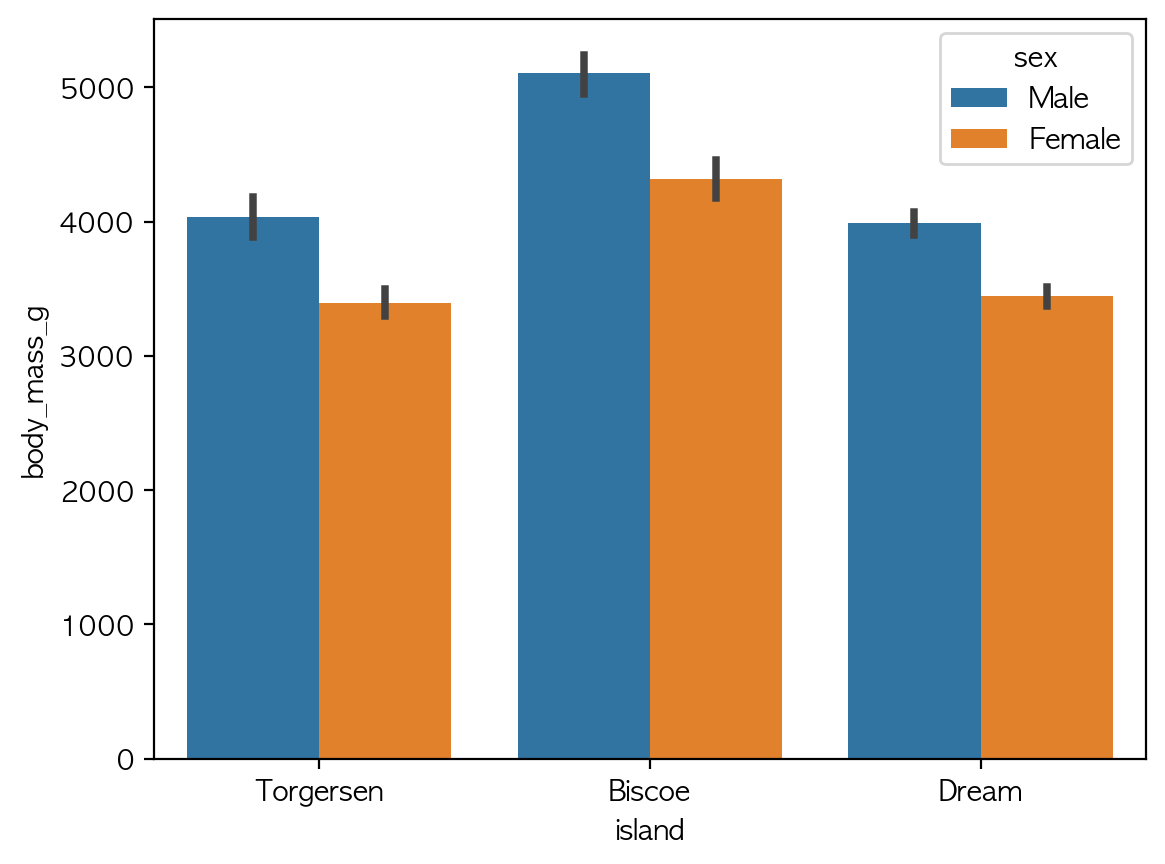
plotly
- 인터렉티브하다.
- 배열, 데이터프레임 가능
In [ ]:
#!pip install plotly
import plotly.express as px
data_canada = px.data.gapminder().query("country == 'Canada'")
data_canada.head()
Out[ ]:
countrycontinentyearlifeExppopgdpPercapiso_alphaiso_num240241242243244
Canada | Americas | 1952 | 68.75 | 14785584 | 11367.16112 | CAN | 124 |
Canada | Americas | 1957 | 69.96 | 17010154 | 12489.95006 | CAN | 124 |
Canada | Americas | 1962 | 71.30 | 18985849 | 13462.48555 | CAN | 124 |
Canada | Americas | 1967 | 72.13 | 20819767 | 16076.58803 | CAN | 124 |
Canada | Americas | 1972 | 72.88 | 22284500 | 18970.57086 | CAN | 124 |
In [ ]:
fig = px.bar(data_canada, x='year', y='pop')
fig.show()
In [ ]:
import plotly.express as px
df = px.data.iris()
df.head()
Out[ ]:
sepal_lengthsepal_widthpetal_lengthpetal_widthspeciesspecies_id01234
5.1 | 3.5 | 1.4 | 0.2 | setosa | 1 |
4.9 | 3.0 | 1.4 | 0.2 | setosa | 1 |
4.7 | 3.2 | 1.3 | 0.2 | setosa | 1 |
4.6 | 3.1 | 1.5 | 0.2 | setosa | 1 |
5.0 | 3.6 | 1.4 | 0.2 | setosa | 1 |
In [ ]:
fig = px.scatter(df, x="sepal_width", y="sepal_length", color="species",
size='petal_length', hover_data=['petal_width'])
fig.show()
In [ ]:
import plotly.graph_objects as go
fig = go.Figure([go.Bar(x=['giraffes', 'orangutans', 'monkeys'], y=[20, 14, 23])])
fig.show()
실습
- '최종주문요일'에 대한 횟수 시각화(마지막으로 주문한 요일의 횟수)
- 성별에 따른 '최종주문요일'에 대한 횟수 시각화(마지막으로 주문한 요일의 횟수)
- '최종주문일자'와 '가입월' 데이터 분포 시각화
- 성별에 따른 '최종주문월'에 대한 '총순수이익' 시각화
- 성별에 따른 나이 분포 시각
- '유입경로'별 '총판매액' 비교
In [ ]:
import pandas as pd
df = pd.read_csv('https://raw.githubusercontent.com/jin0choi1216/dataset/main/%40preprocessing_data_member01.csv', index_col = 0)
df.head()
Out[ ]:
ID총구매수량_x기타품목구매비율수저류구매비율실링구매비율용기류구매비율위생용품구매비율총구매횟수총판매액총할인금액...최종주문연도최종주문월최종주문일자최종주문요일최종주문시간회원가입일(clean)가입연도가입월가입일자가입요일01234
100304734@n | 1.0 | 0.0 | 0.0 | 0.0 | 1.0 | 0.0 | 1 | 103300 | 0 | ... | 2019.0 | 5.0 | 9.0 | Thursday | 14.0 | 2019-05-09 00:00:00 | 2019.0 | 5.0 | 9.0 | Thursday |
1003409866@k | 62.0 | 0.0 | 0.0 | 0.0 | 1.0 | 0.0 | 18 | 4303880 | 379040 | ... | 2019.0 | 5.0 | 28.0 | Tuesday | 16.0 | 2019-01-07 00:00:00 | 2019.0 | 1.0 | 7.0 | Monday |
100381931@n | 5.0 | 0.0 | 0.0 | 0.0 | 0.0 | 1.0 | 3 | 71050 | 15000 | ... | 2019.0 | 2.0 | 10.0 | Sunday | 21.0 | 2018-10-31 00:00:00 | 2018.0 | 10.0 | 31.0 | Wednesday |
1004498382@k | 1.0 | 0.0 | 0.0 | 0.0 | 1.0 | 0.0 | 1 | 76400 | 14500 | ... | 2019.0 | 1.0 | 9.0 | Wednesday | 12.0 | 2019-01-09 00:00:00 | 2019.0 | 1.0 | 9.0 | Wednesday |
1004547839@k | 4.0 | 1.0 | 0.0 | 0.0 | 0.0 | 0.0 | 1 | 360800 | 53400 | ... | 2019.0 | 1.0 | 9.0 | Wednesday | 13.0 | 2019-01-09 00:00:00 | 2019.0 | 1.0 | 9.0 | Wednesday |
5 rows × 35 columns
In [ ]:
df.columns
Out[ ]:
Index(['ID', '총구매수량_x', '기타품목구매비율', '수저류구매비율', '실링구매비율', '용기류구매비율', '위생용품구매비율',
'총구매횟수', '총판매액', '총할인금액', '총순수이익', '나이', '사용가능 적립금', '성별', '최종접속일',
'최종주문일', '유입경로', '유입기기', '회원 가입일', '사업자구분', '회원구분', '지역', '세부지역', '주소',
'최종주문일(clean)', '최종주문연도', '최종주문월', '최종주문일자', '최종주문요일', '최종주문시간',
'회원가입일(clean)', '가입연도', '가입월', '가입일자', '가입요일'],
dtype='object')
1. '최종주문요일'에 대한 횟수 시각화(마지막으로 주문한 요일의 횟수)
In [ ]:
df1 = df.groupby(['최종주문요일']).size().sort_values(ascending=False)
df1.index
Out[ ]:
Index(['Monday', 'Wednesday', 'Tuesday', 'Thursday', 'Friday', 'Sunday',
'Saturday'],
dtype='object', name='최종주문요일')
In [ ]:
import matplotlib.pyplot as plt
fig, ax = plt.subplots()
최종주문요일 = df1.index
counts = df1.values
#bar_labels = ['red', 'blue', '_red', 'orange']
#bar_colors = ['tab:red', 'tab:blue', 'tab:red', 'tab:orange']
ax.bar(최종주문요일, counts)#, label=bar_labels, color=bar_colors)
ax.set_ylabel('주문횟수')
ax.set_title('마지막으로 주문한 요일의 횟수')
ax.legend(title='요일')
plt.show()
No artists with labels found to put in legend. Note that artists whose label start with an underscore are ignored when legend() is called with no argument.
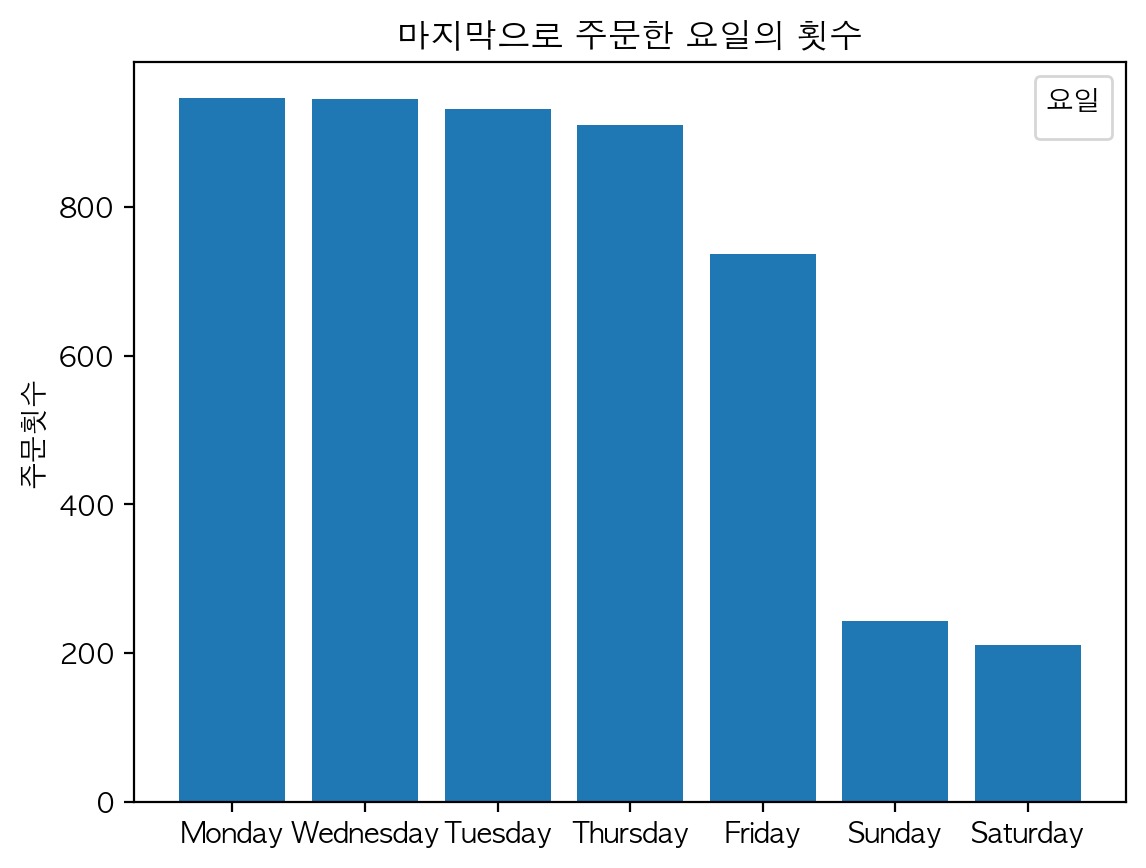
In [ ]:
# 1. '최종주문요일'에 대한 주문횟수 시각화(마지막으로 주문한 각 요일의 횟수)
import seaborn as sns
q1 = df['최종주문요일'].value_counts().to_frame()
sns.barplot(data=q1, x=q1.index, y='count')
Out[ ]:
<Axes: xlabel='최종주문요일', ylabel='count'>
In [ ]:
sns.countplot(x=df["최종주문요일"])
Out[ ]:
<Axes: xlabel='최종주문요일', ylabel='count'>
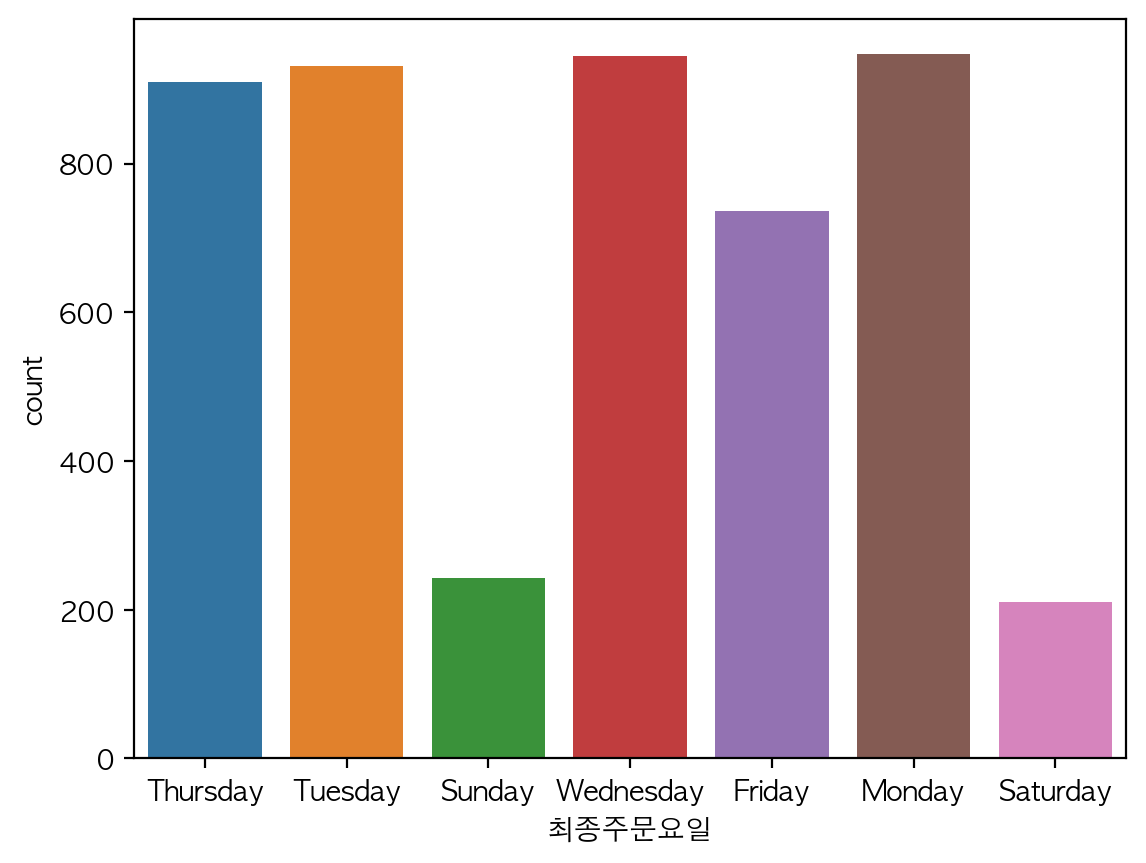
In [ ]:
import matplotlib.pyplot as plt
d1 = df[['최종주문요일','총구매횟수']].groupby('최종주문요일').count().sort_values('최종주문요일')
plt.bar(d1.index, d1['총구매횟수'])
Out[ ]:
<BarContainer object of 7 artists>
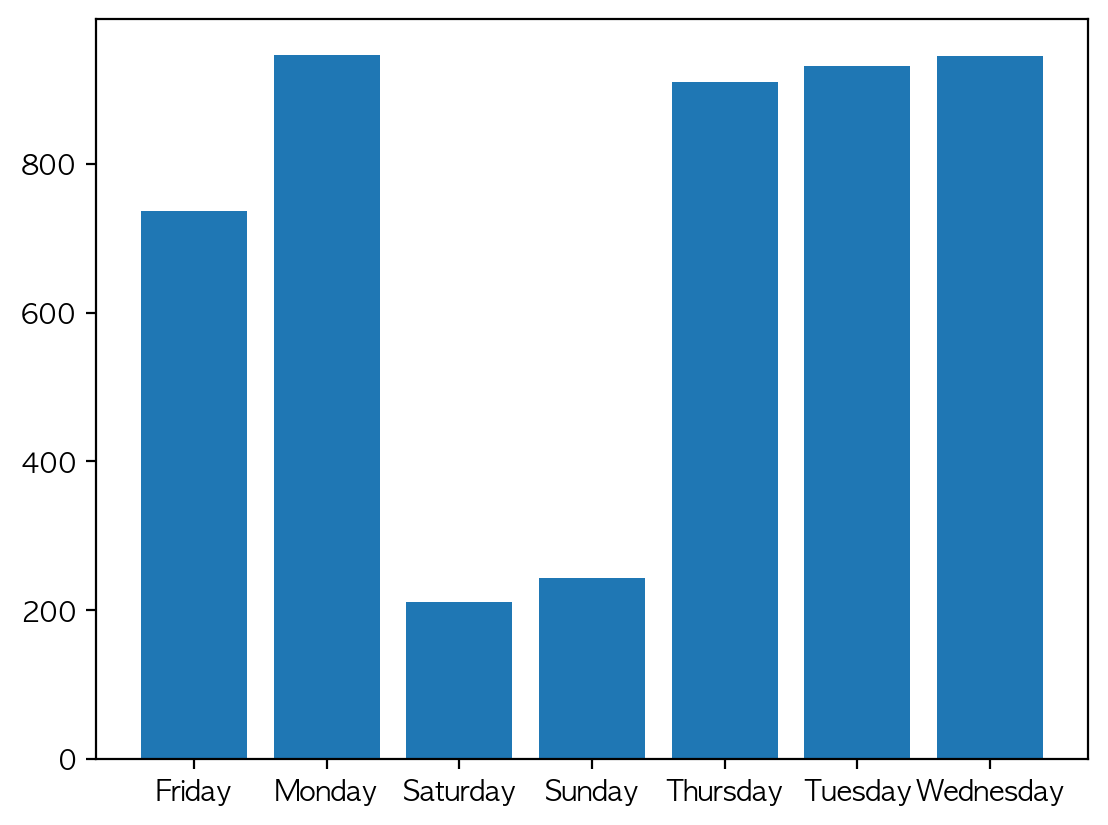
2. 성별에 따른 '최종주문요일'에 대한 횟수 시각화(마지막으로 주문한 요일의 횟수)
In [ ]:
from os import name
df2 = df[['성별','최종주문요일']]
df2_m = df2.query('성별 == "남자"').groupby('최종주문요일').count()
df2_f = df2.query('성별 == "여자"').groupby('최종주문요일').count()
df2 = pd.merge(df2_m, df2_f, on='최종주문요일')
df2_m
# df2_total = df2
Out[ ]:
성별최종주문요일FridayMondaySaturdaySundayThursdayTuesdayWednesday
676 |
871 |
197 |
222 |
850 |
861 |
883 |
In [ ]:
# data from https://allisonhorst.github.io/palmerpenguins/
import matplotlib.pyplot as plt
import numpy as np
species = df2.index
#penguin_means = df2
x = np.arange(len(species)) # the label locations
width = 0.2 # the width of the bars
multiplier = 0
fig, ax = plt.subplots(layout='constrained')
for attribute, counts in df2.items():
offset = width * multiplier
rects = ax.bar(x + offset, counts, width, label=attribute)
ax.bar_label(rects, padding=3)
multiplier += 1
# Add some text for labels, title and custom x-axis tick labels, etc.
ax.set_ylabel('Count')
ax.set_title('성별에 따른 최종주문요일에 대한 횟수')
ax.set_xticks(x + width, species)
ax.legend(loc='upper left', ncols=3)
ax.set_ylim(0, 250)
plt.show()
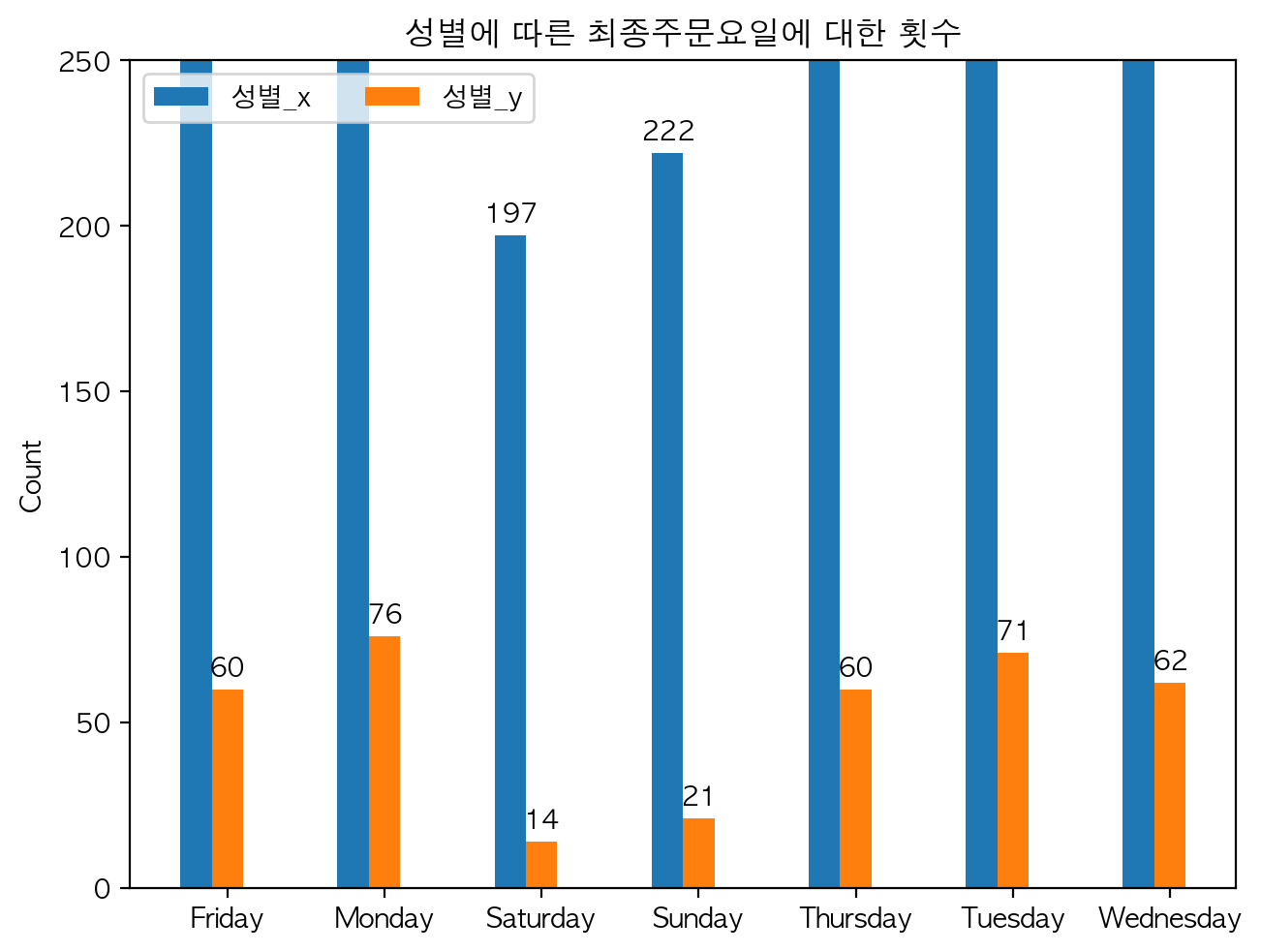
In [ ]:
# 2. 성별에 따른 '최종주문요일'에 대한 횟수 시각화(마지막으로 주문한 요일의 횟수)
q1 = df[['성별','최종주문요일','ID']].groupby(['성별', '최종주문요일'], as_index = False).count()
q1.rename(columns = {'ID' : 'user_count'}, inplace = True)
sns.barplot(data=q1, x="최종주문요일", y="user_count", hue="성별")
Out[ ]:
<Axes: xlabel='최종주문요일', ylabel='user_count'>
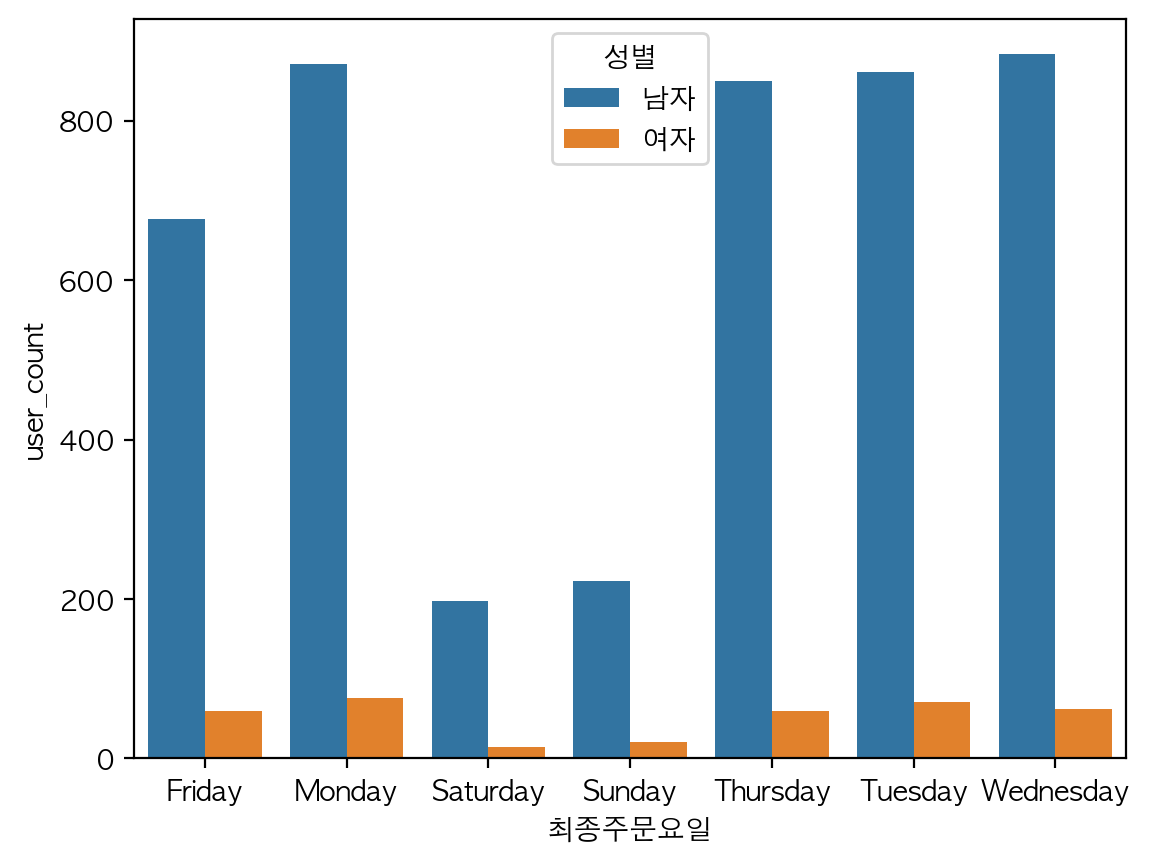
3. '최종주문일자'와 '가입월' 데이터 분포 시각화
In [ ]:
#!pip install nbformat
In [ ]:
# 3. '최종주문일자'와 '가입월' 데이터 분포 시각화
import plotly.express as px
fig = px.histogram(df, x="최종주문일자")
fig.show()
In [ ]:
fig = px.histogram(df, x="가입월")
fig.show()
4. 성별에 따른 '최종주문월'에 대한 '총순수이익' 시각화
In [ ]:
# 4. 성별에 따른 '최종주문월'에 대한 '총순수이익' 시각화
sns.lineplot(data=df, x="최종주문월", y="총순수이익", hue="성별")
Out[ ]:
<Axes: xlabel='최종주문월', ylabel='총순수이익'>
In [ ]:
sns.barplot(data=df, x="최종주문월", y="총순수이익", hue="성별")
Out[ ]:
<Axes: xlabel='최종주문월', ylabel='총순수이익'>
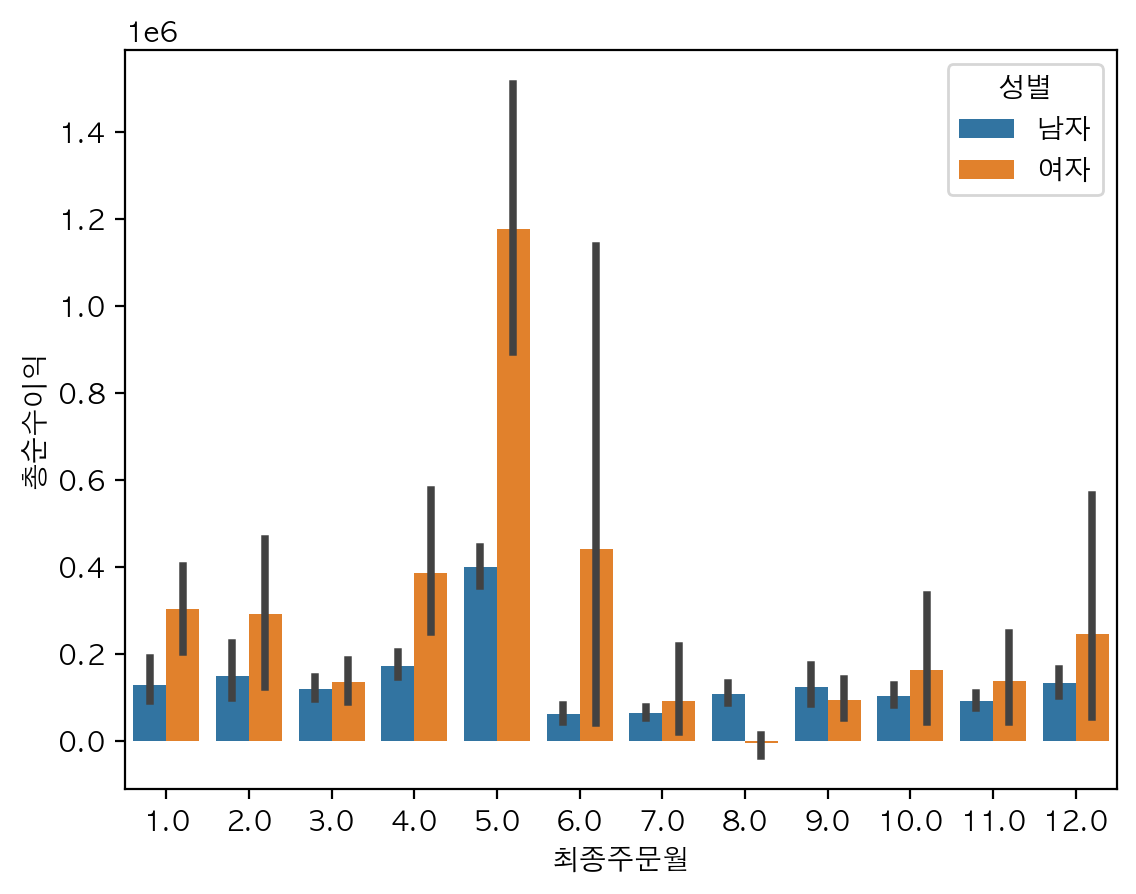
5. 성별에 따른 나이 분포 시각
In [ ]:
df[['성별', '나이']]
Out[ ]:
성별나이01234...49314932493349344935
남자 | 47.0 |
남자 | 27.0 |
남자 | 41.0 |
남자 | 47.0 |
남자 | 47.0 |
... | ... |
남자 | 45.0 |
남자 | 32.0 |
여자 | 40.0 |
남자 | 45.0 |
남자 | 46.0 |
4924 rows × 2 columns
In [ ]:
import plotly.express as px
fig = px.violin(df, y="나이", color="성별")
fig.show()
6. '유입경로'별 '총판매액' 비교
In [ ]:
df[['유입경로', '총판매액']]
plt.figure(figsize = (12,5))
sns.barplot(data=df, x='유입경로', y='총판매액')
Out[ ]:
<Axes: xlabel='유입경로', ylabel='총판매액'>
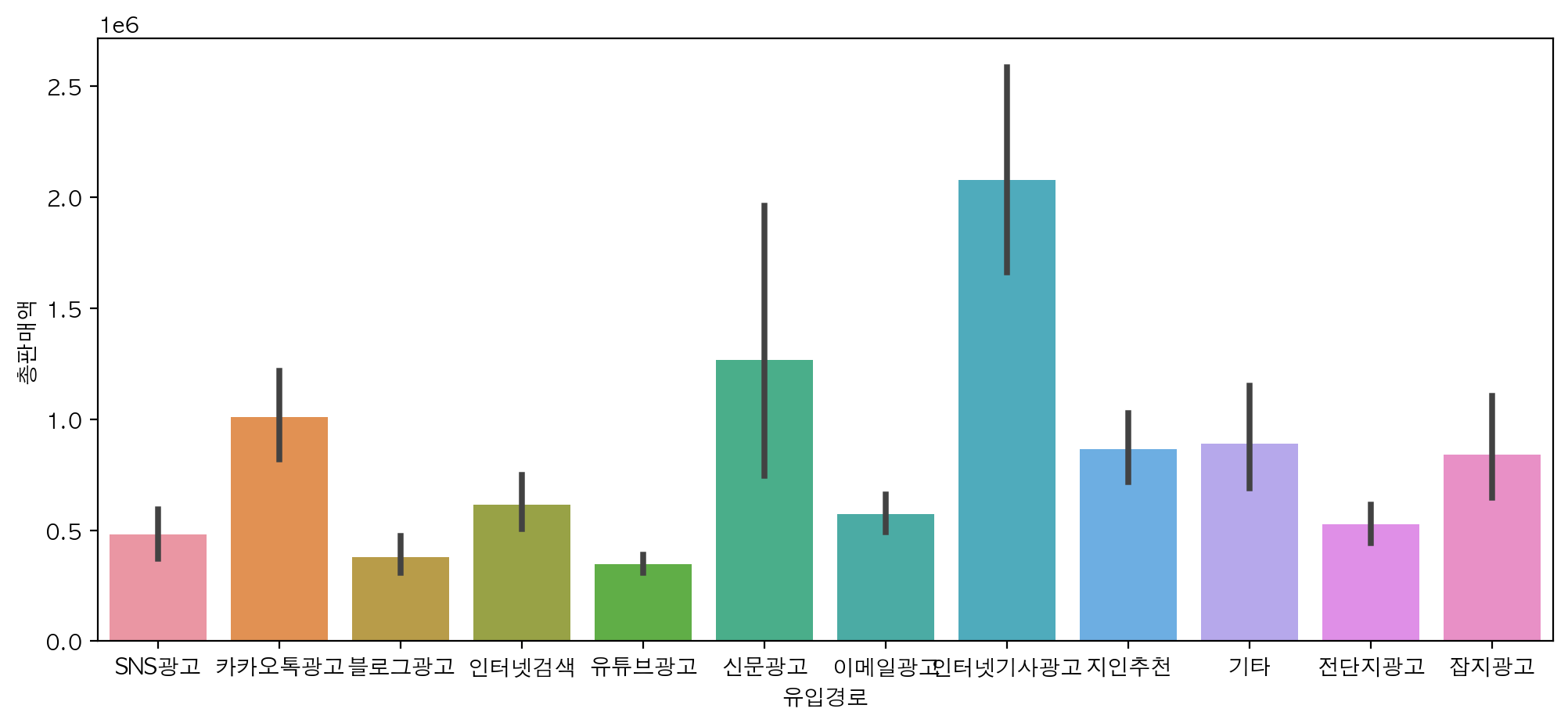
과제
데이터셋 & 샘플
In [ ]:
df = pd.read_csv('0914_shopping_mall.csv',index_col=0)
df['price'] = df['item_price'] * df['quantity']
df.head()
Out[ ]:
detail_idtransaction_iditem_idquantitypayment_datecustomer_idcustomer_nameregistration_dateemailgenderagebirthprefitem_nameitem_priceprice01234
0 | T0000000113 | S005 | 1 | 2019-02-01 01:36:57 | PL563502 | 김태경 | 2019-01-07 14:34 | imoto_yoshimasa@example.com | M | 30 | 1989-07-15 | 대전광역시 | PC-E | 210000 | 210000 |
1 | T0000000114 | S001 | 1 | 2019-02-01 01:37:23 | HD678019 | 김영웅 | 2019-01-27 18:00 | mifune_rokurou@example.com | M | 73 | 1945-11-29 | 서울특별시 | PC-A | 50000 | 50000 |
2 | T0000000115 | S003 | 1 | 2019-02-01 02:34:19 | HD298120 | 김강현 | 2019-01-11 8:16 | yamane_kogan@example.com | M | 42 | 1977-05-17 | 광주광역시 | PC-C | 120000 | 120000 |
3 | T0000000116 | S005 | 1 | 2019-02-01 02:47:23 | IK452215 | 김주한 | 2019-01-10 5:07 | ikeda_natsumi@example.com | F | 47 | 1972-03-17 | 인천광역시 | PC-E | 210000 | 210000 |
4 | T0000000117 | S002 | 2 | 2019-02-01 04:33:46 | PL542865 | 김영빈 | 2019-01-25 6:46 | kurita_kenichi@example.com | M | 74 | 1944-12-17 | 광주광역시 | PC-B | 85000 | 170000 |
In [ ]:
# 지역별 매출?
q1 =df[['pref','price']].\
groupby('pref',as_index=False).\
sum().sort_values(by='price',ascending=False)
# ,as_index=False -> 그래프 그릴 때 인덱스 사용
In [ ]:
import plotly.express as px
fig = px.bar(q1, x='pref',y='price', color = 'pref')
fig.show()
In [ ]:
fig = px.pie(df, values='price', names='pref', title='지역별 매출?')
fig.update_traces(textposition='inside', textinfo='label+percent')
fig.show()
In [ ]:
# 성별 상품 선호도?
q2 = df[['gender', 'quantity', 'item_name']]
q2g = q2.groupby(['item_name', 'gender'], as_index = False).sum()
In [ ]:
fig = px.bar(q2g, x="item_name", y="quantity", color="gender", barmode="group")
fig.show()
1. 나이 별 상품 선호도?
In [ ]:
df = pd.read_csv('0914_shopping_mall.csv',index_col=0)
df['price'] = df['quantity']*df['item_price']
df['payment_date'] = pd.to_datetime(df['payment_date'],format='%Y-%m-%d %H:%M:%S')
df.head(2)
Out[ ]:
detail_idtransaction_iditem_idquantitypayment_datecustomer_idcustomer_nameregistration_dateemailgenderagebirthprefitem_nameitem_priceprice01
0 | T0000000113 | S005 | 1 | 2019-02-01 01:36:57 | PL563502 | 김태경 | 2019-01-07 14:34 | imoto_yoshimasa@example.com | M | 30 | 1989-07-15 | 대전광역시 | PC-E | 210000 | 210000 |
1 | T0000000114 | S001 | 1 | 2019-02-01 01:37:23 | HD678019 | 김영웅 | 2019-01-27 18:00 | mifune_rokurou@example.com | M | 73 | 1945-11-29 | 서울특별시 | PC-A | 50000 | 50000 |
In [ ]:
df1 = df[['gender','item_name','quantity']]\
.groupby(['gender','item_name'], as_index=False)\
.sum()
df1
Out[ ]:
genderitem_namequantity0123456789
F | PC-A | 1526 |
F | PC-B | 879 |
F | PC-C | 520 |
F | PC-D | 445 |
F | PC-E | 893 |
M | PC-A | 1517 |
M | PC-B | 906 |
M | PC-C | 502 |
M | PC-D | 455 |
M | PC-E | 929 |
In [ ]:
import plotly.express as px
fig = px.bar(df1, x="item_name", y="quantity", color="gender", barmode="group")
fig.show()
2. 지역별 성별 당 총 소비 가격
In [ ]:
df2 = df[['gender', 'pref','price']].groupby(['gender', 'pref'], as_index=False).sum()
df2
Out[ ]:
genderprefprice012345678910111213
F | 광주광역시 | 43085000 |
F | 대구광역시 | 48710000 |
F | 대전광역시 | 85450000 |
F | 부산광역시 | 61130000 |
F | 서울특별시 | 98725000 |
F | 울산광역시 | 40620000 |
F | 인천광역시 | 103325000 |
M | 광주광역시 | 38855000 |
M | 대구광역시 | 52080000 |
M | 대전광역시 | 97755000 |
M | 부산광역시 | 64105000 |
M | 서울특별시 | 89060000 |
M | 울산광역시 | 44030000 |
M | 인천광역시 | 104205000 |
In [ ]:
fig = px.bar(df2, x="pref", y="price", color="gender", barmode="group")
fig.show()
In [ ]:
# https://youngwonhan-family.tistory.com/entry/%EA%B5%AD%EB%82%B4-%EC%8B%9C%EB%8F%84%EB%B3%84-%EC%BD%94%EB%A1%9C%EB%82%98-19-%ED%99%95%EC%A7%84-%EC%A0%95%EB%B3%B4-Plotly-%EA%B3%B5%EA%B0%84%EC%A0%95%EB%B3%B4-%EB%8B%A8%EA%B3%84%EA%B5%AC%EB%B6%84%EB%8F%84-choropleth-map
import json
with open('korea_geojson2.geojson', encoding='UTF-8') as f:
data = json.load(f)
for x in data['features']:
x['id'] = x['properties']['CTP_KOR_NM']
for idx, _ in enumerate(data['features']):
print(data['features'][idx]['id'])
강원도
경기도
경상남도
경상북도
광주광역시
대구광역시
대전광역시
부산광역시
서울특별시
세종특별자치시
울산광역시
인천광역시
전라남도
전라북도
제주특별자치도
충청남도
충청북도
In [ ]:
df2_m = df2.query('gender == "M"')
df2_f = df2.query('gender == "F"')
df2_m
Out[ ]:
genderprefprice78910111213
M | 광주광역시 | 38855000 |
M | 대구광역시 | 52080000 |
M | 대전광역시 | 97755000 |
M | 부산광역시 | 64105000 |
M | 서울특별시 | 89060000 |
M | 울산광역시 | 44030000 |
M | 인천광역시 | 104205000 |
In [ ]:
fig = px.choropleth_mapbox(
df2_m,
geojson=data,
locations='pref',
color='price',
color_continuous_scale=px.colors.sequential.Redor,
mapbox_style="carto-positron",
zoom=5.5,
center = {"lat": 35.757981, "lon": 127.661132},
opacity=0.6,
labels={'price':'남성 소비 금액'}
)
fig.update_layout(margin={"r":0,"t":0,"l":0,"b":0})
fig.show()
In [ ]:
fig = px.choropleth_mapbox(
df2_f,
geojson=data,
locations='pref',
color='price',
color_continuous_scale=px.colors.sequential.Redor,
mapbox_style="carto-positron",
zoom=5.5,
center = {"lat": 35.757981, "lon": 127.661132},
opacity=0.6,
labels={'price':'여성 소비 금액'}
)
fig.update_layout(margin={"r":0,"t":0,"l":0,"b":0})
fig.show()
3. 월별 구매량
In [ ]:
df.head(2)
Out[ ]:
detail_idtransaction_iditem_idquantitypayment_datecustomer_idcustomer_nameregistration_dateemailgenderagebirthprefitem_nameitem_priceprice01
0 | T0000000113 | S005 | 1 | 2019-02-01 01:36:57 | PL563502 | 김태경 | 2019-01-07 14:34 | imoto_yoshimasa@example.com | M | 30 | 1989-07-15 | 대전광역시 | PC-E | 210000 | 210000 |
1 | T0000000114 | S001 | 1 | 2019-02-01 01:37:23 | HD678019 | 김영웅 | 2019-01-27 18:00 | mifune_rokurou@example.com | M | 73 | 1945-11-29 | 서울특별시 | PC-A | 50000 | 50000 |
In [ ]:
import datetime
# df3 = df['payment_date'].dt.strftime('%Y%m').rename('payment_month')
# df3
df3 = pd.concat([df,
df['payment_date'].dt.strftime('%Y%m').
rename('payment_month')],axis=1)
df3.head(2)
Out[ ]:
detail_idtransaction_iditem_idquantitypayment_datecustomer_idcustomer_nameregistration_dateemailgenderagebirthprefitem_nameitem_pricepricepayment_month01
0 | T0000000113 | S005 | 1 | 2019-02-01 01:36:57 | PL563502 | 김태경 | 2019-01-07 14:34 | imoto_yoshimasa@example.com | M | 30 | 1989-07-15 | 대전광역시 | PC-E | 210000 | 210000 | 201902 |
1 | T0000000114 | S001 | 1 | 2019-02-01 01:37:23 | HD678019 | 김영웅 | 2019-01-27 18:00 | mifune_rokurou@example.com | M | 73 | 1945-11-29 | 서울특별시 | PC-A | 50000 | 50000 | 201902 |
In [ ]:
df3[['payment_month','price']].head(2)
Out[ ]:
payment_monthprice01
201902 | 210000 |
201902 | 50000 |
In [ ]:
import plotly.express as px
fig = px.scatter(df3, x="payment_month", y="price"
, marginal_x="histogram", marginal_y="rug")
fig.show()
4. 성씨별 구매력?..
5. 품목별 구매량?
6. 가장 매출이 좋은 시간대? 날짜?
7. 나이 성별 분포
728x90
'새싹 > TIL' 카테고리의 다른 글
[핀테커스] 230918 데이터 시각화 라이브러리 실습 (1) | 2023.09.18 |
---|---|
[핀테커스] 230915 미니프로젝트 (0) | 2023.09.15 |
[핀테커스] 230913 pandas merge & metplot 시각화 (0) | 2023.09.13 |
[핀테커스] 230912 pandas & 시계열데이터다루기 (0) | 2023.09.12 |
[핀테커스] 230911 데이터 사이언스 라이브러리 pandas 실습 (0) | 2023.09.11 |